Transit Module 22: Harnessing Social Media and Big Data Technologies for Transit Business Intelligence
HTML of the PowerPoint Presentation
(Note: This document has been converted from a PowerPoint presentation to 508-compliant HTML. The formatting has been adjusted for 508 compliance, but all the original text content is included, plus additional text descriptions for the images, photos and/or diagrams have been provided below.)
Slide 1:
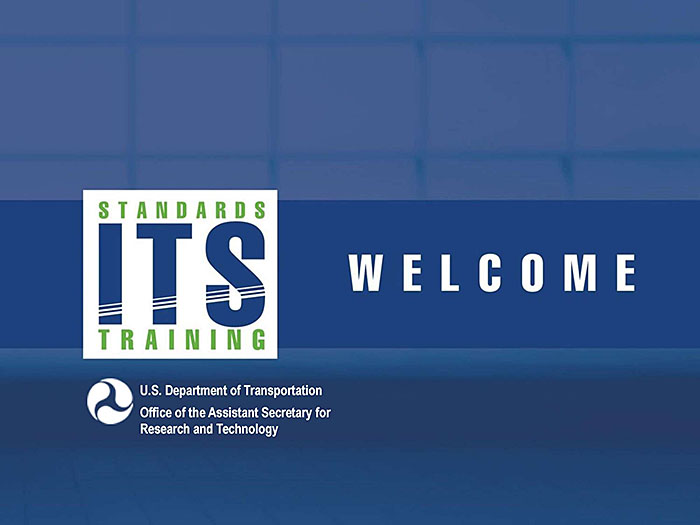
Slide 2:
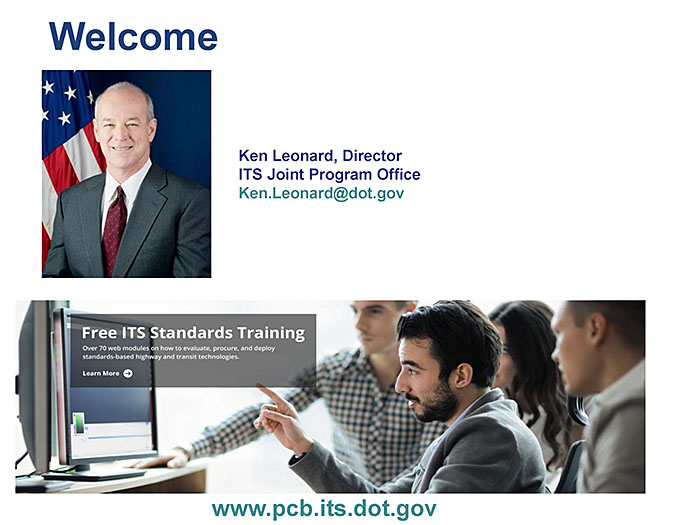
Slide 3:
Module 22:
Harnessing Social Media & Big Data Technologies for Transit Business Intelligence
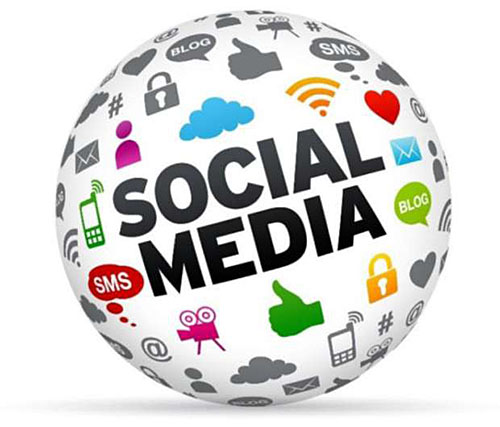
Slide 4:
Instructor
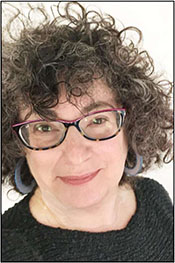
Susan Bregman Principal
Oak Square Resources, LLC
Slide 5:
Instructor
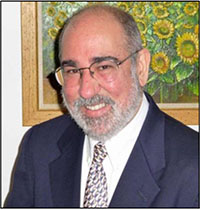
Manny Insignares
Vice President Technology
Consensus Systems Technologies
Slide 6:
Learning Objectives
- Define how transit providers use business intelligence
- Define social media platforms and their applications to public transportation
- Define big data in relation to social media and transit
- Understand the process for applying big data analytics to social media to inform transit business intelligence
- Incorporate findings to support business intelligence with data-driven decisions
Slide 7:
Learning Objective 1
- Define how transit providers use business intelligence
Slide 8:
Overview
- What is business intelligence?
- What are potential data sources?
- How can business intelligence benefit transit operators?
Slide 9:
What is Business Intelligence?
-
Combines information from multiple sources to support data-driven decisions
- Quantitative - Ridership, fare revenue, mileage
- Qualitative - Focus groups, interviews, social media
-
Integrates data from internal and external sources
- Internal - Automated vehicle location systems, customer panels
- External - Social media posts, Census data
- Enables organizations to evaluate progress in achieving goals
- Supports internal decision-making
Slide 10:
What are Potential Data Sources?
-
Qualitative data (agency-generated)
- Customer surveys and panels
- Focus groups and stakeholder interviews
-
Quantitative data (agency-generated)
- Automatic passenger counting data (APC)
- Automated vehicle location data (AVL)
- General Transit Feed Specification files (GTFS and GTFS-rt)
- Electronic fare payment system datasets (EFPS)
-
External data sources
- Social media posts
- Census files and other public datasets
Slide 11:
How Can Business Intelligence Benefit Transit Operators?
- Meet mandated reporting requirements
- Provide greater transparency in reporting to internal and external audiences
- Provide input for planning, operations, and capital investments
- Support briefings for senior staff and board of directors
Slide 12:
How Can Business Intelligence Benefit Transit Operators?
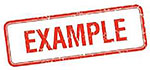

GOAL
Improve customer satisfaction ACTIONS
- Conduct surveys and focus groups.
- Establish online customer panel.
- Analyze social media posts to understand customer sentiment.
Slide 13:
How Can Business Intelligence Benefit Transit Operators?
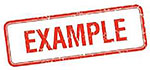
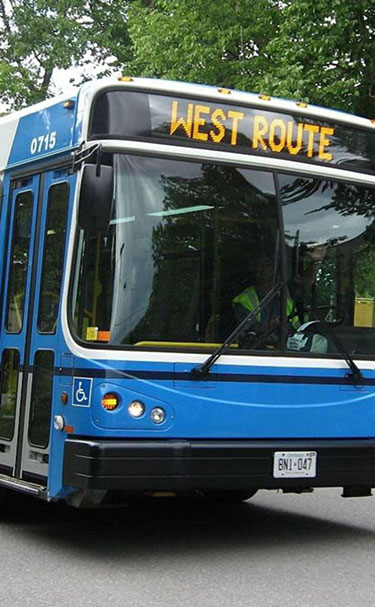
GOAL
Improve service reliability for bus operations
ACTIONS
- Review internal data on on-time performance and travel time.
- Examine social media posts to identify specific locations where bus routes are prone to delay.
Slide 14:
How Can Business Intelligence Benefit Transit Operators?
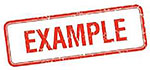
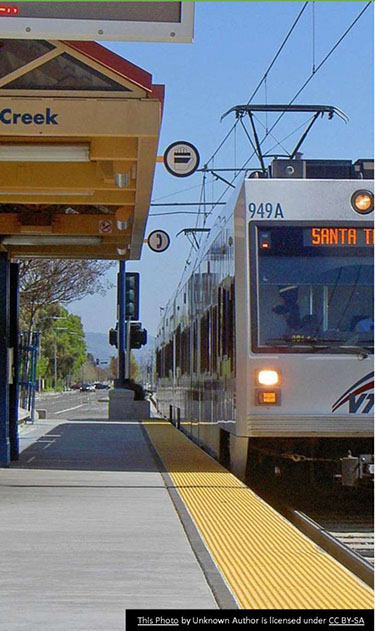
GOAL
Improve maintenance at rail stations ACTIONS
- Review internal maintenance records.
- Analyze social media posts to identify issues on specific vehicles or stations.
- Encourage customers to report issues via social media (e.g., broken lights, overflowing trash, disabled ticketing machines).
Slide 15:
How Can Business Intelligence Benefit Transit Operators?
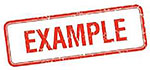
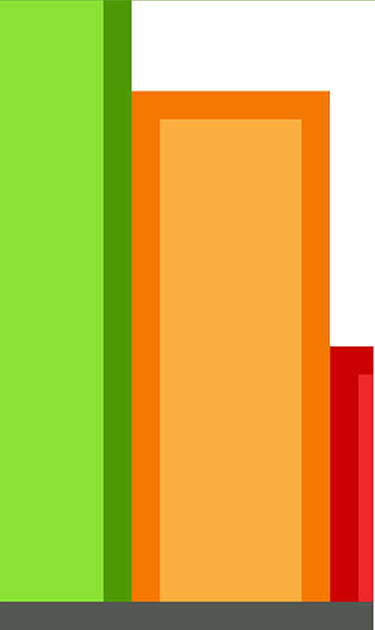
GOAL
Improve transparency in performance reporting.
ACTIONS
- Develop key performance indicators (KPI) from available data sources.
- Report KPIs via online performance dashboard.
Slide 16:

Slide 17:
Question
Which of the following is NOT a source of data for business intelligence?
Answer Choices
- Automatic passenger counters (APC)
- Social media posts
- Electronic fare collection systems (EFCS)
- None of the above
Slide 18:
Review of Answers
a) Automatic passenger counters (APC)
Incorrect. APC data can be analyzed to support agency decision-making.
b) Social media posts
Incorrect. Social media posts can be analyzed to support agency decision-making.
c) Electronic fare collection systems data (EFCS)
Incorrect. EFCS data can be analyzed to support agency decision-making.
d) None of the above
Correct! All the data sources listed can be used to support transit decision-making.
Slide 19:
Learning Objective 2
- Define social media platforms and their applications to public transportation
Slide 20:
Overview
- What is social media?
- Taxonomy of social media platforms
- Use of social media by transit operators for agency-generated information
- Use of social media by transit customers and stakeholders for user-generated information
- Use of crowdsourcing and peer-to-peer platforms for sharing communication about transit
Slide 21:
What is Social Media?

- Social media platforms are web-based or mobile applications that encourage users to interact with (and often influence) one another in real time.
- Social media, also called social networking, includes different types of applications.
- Platforms are mostly owned by private companies with proprietary formats and are not consistently regulated.
- Social media posts can share information (and misinformation).
- Social media is still evolving, and platforms continue to change.
Slide 22:
Taxonomy of Social Media
- Social networks
- Media sharing networks
- Discussion forums
- Content curation
- Consumer review networks
- Blogging and publishing networks
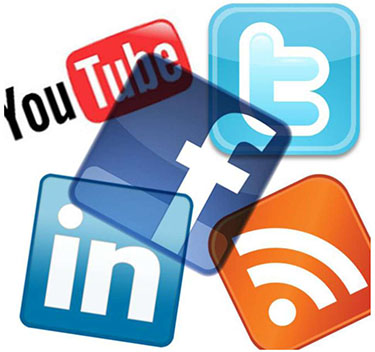
Slide 23:
Taxonomy of Social Media
Social Networks
- Connect with other people online
- Share information, comments, and media
- Personal and professional networks
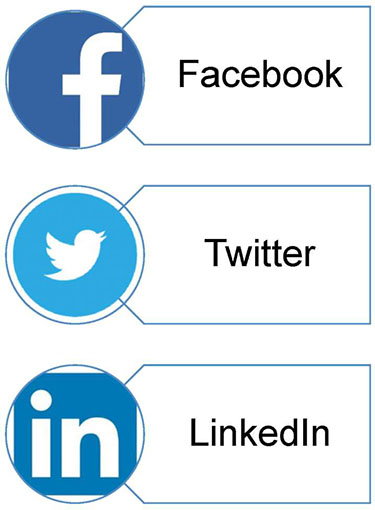
Slide 24:
Taxonomy of Social Media
Media-Sharing Networks
- Share images, videos, and other types of media with others.
- Offer comments and other forms of feedback.
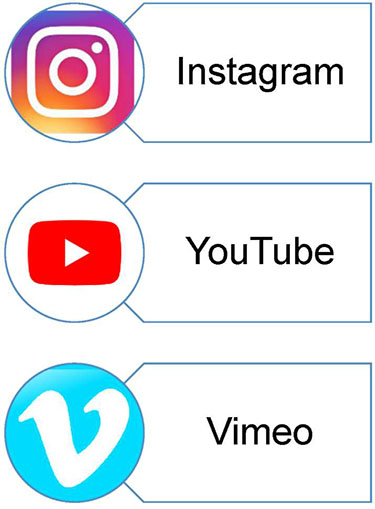
Slide 25:
Taxonomy of Social Media
Discussion Forums
- Platforms serve as discussion boards
- Users can ask and answer questions, share information, and participate in discussions
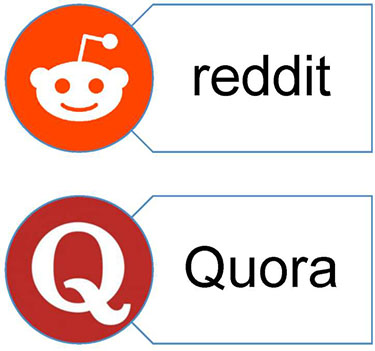
Slide 26:
Taxonomy of Social Media
Content Curation Platforms
- Identify and share content from multiple sources
- Content types include photographs, graphics, videos, presentations, and text
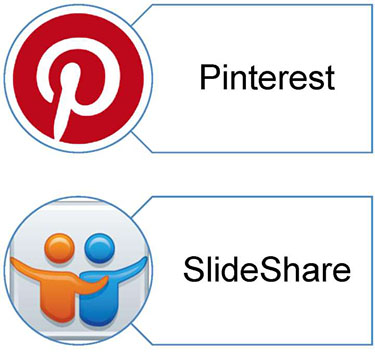
Slide 27:
Taxonomy of Social Media
Consumer Review Networks
- Generate reviews and share opinions about goods and services.
- Most consumer websites also include customer reviews (e.g., Amazon).
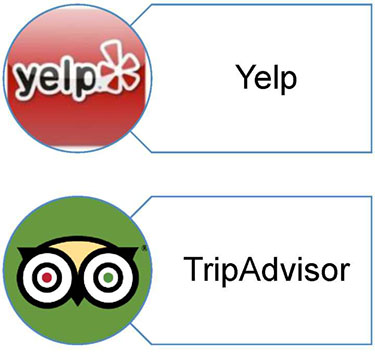
Slide 28:
Taxonomy of Social Media
Blogging and Publishing Networks
- Create content on user-defined topics.
- Posts are typically longer than most social networking sites.
- Organizations may use platforms to share news.
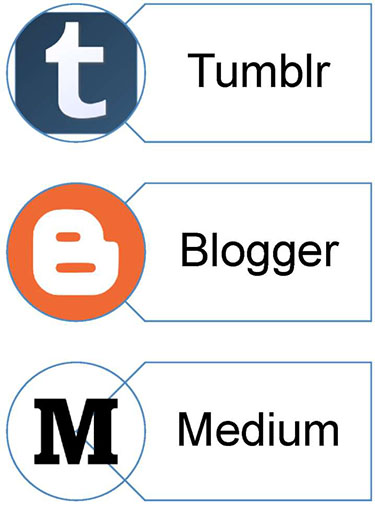
Slide 29:
Agency-Generated Social Media
Overview
-
Most transit operators use social media for outbound communications.
- Service updates and alerts
- Emergency communications
- Marketing activities
- Customer service
- Solicit customer feedback
- General agency communications
- Audiences may include riders, stakeholders, media, first responders, public officials, and community members.

Slide 30:
Agency-Generated Social Media
Service Updates and Alerts
-
Notify customers about service changes
- Provide information about traffic delays and construction impacts
- Provide details about service during special events
- Twitter is especially well-suited for real-time alerts
Slide 31:
Agency-Generated Social Media
Service Updates and Alerts

Slide 32:
Agency-Generated Social Media
Emergency Communications
- Use social media to communicate during health emergencies, weather events, and natural disasters (e.g., COVID-19, hurricanes, earthquakes).
- Use social media to share public safety information (e.g., Amber alerts, criminal activity).
- Twitter is especially well-suited for real-time alerts.
Slide 33:
Agency-Generated Social Media
COVID-19 Pandemic Communications
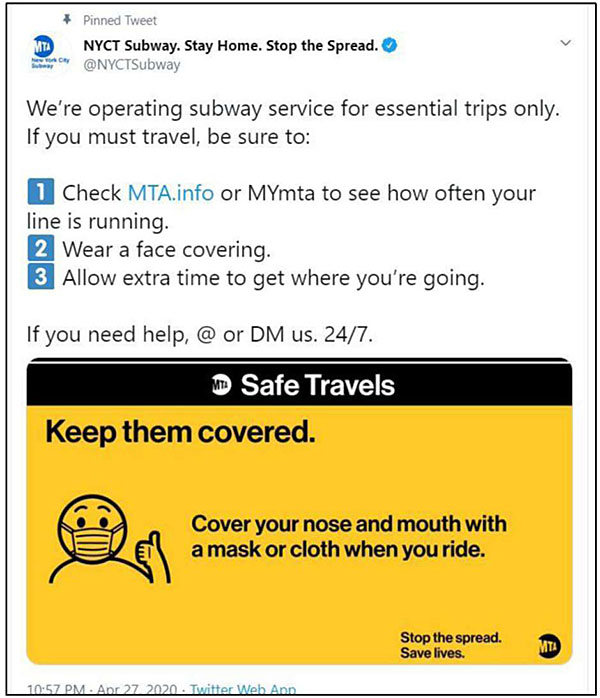
Slide 34:
Agency-Generated Social Media
Public Safety Communications
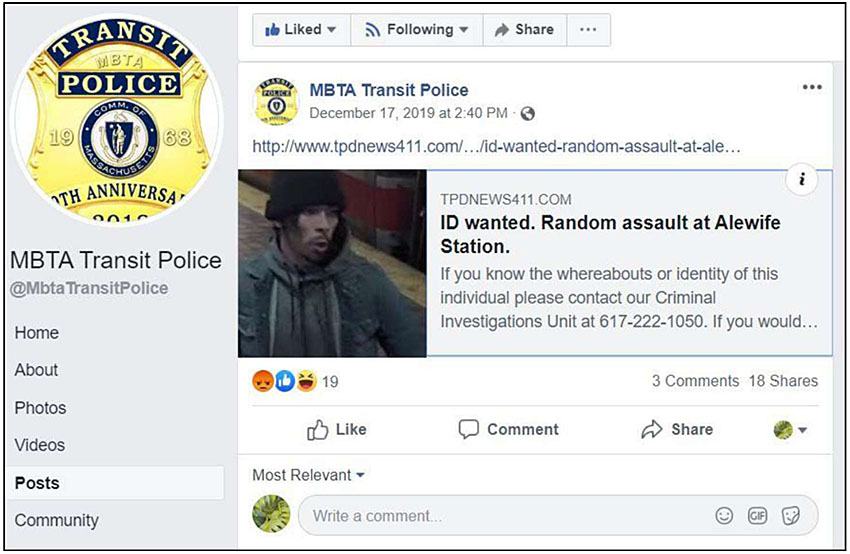
Slide 35:
Agency-Generated Social Media
Marketing Activities
- Social media can help agencies create an image or identity.
- Media-sharing and blogging platforms are a good match for these posts.
Slide 36:
Agency-Generated Social Media
Marketing Activities

Slide 37:
Agency-Generated Social Media
Customer Service
- Provide real-time customer service.
- Address customer comments and complaints.
Slide 38:
Agency-Generated Social Media
Customer Service
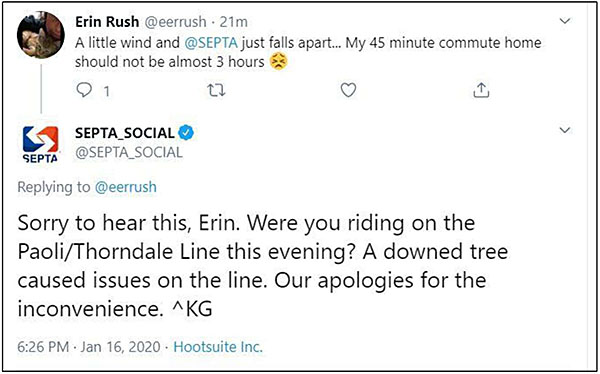
Slide 39:
Agency-Generated Social Media
Solicit Customer Feedback
- Use social media to reach out to customers.
- Seek feedback on projects or programs.
Slide 40:
Agency-Generated Social Media
Solicit Customer Feedback
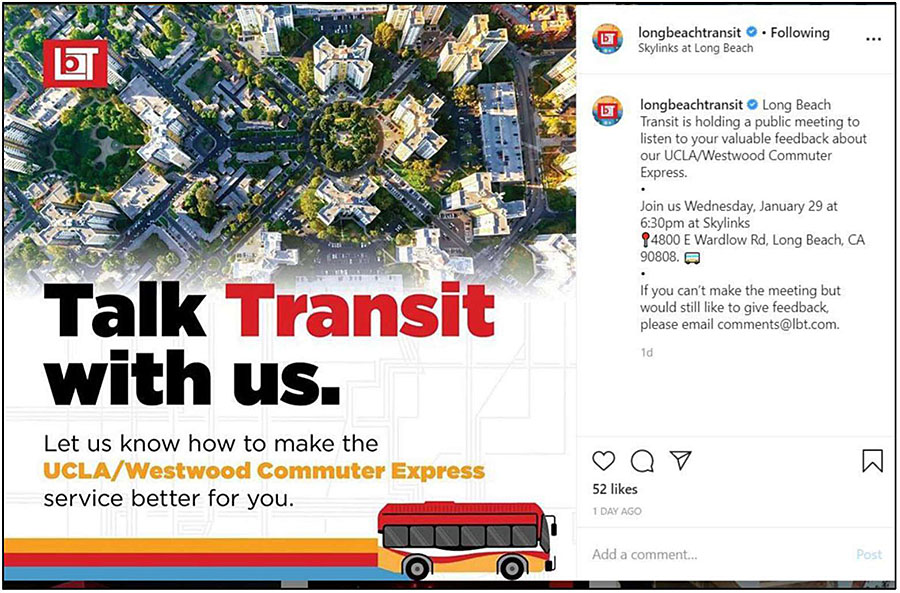
Slide 41:
Agency-Generated Social Media
General Agency Announcements
- Share agency information
- Job listings
- Press releases
- Social posts can complement - but should not replace - traditional communications channels.
Slide 42:
Agency-Generated Social Media
General Agency Announcements
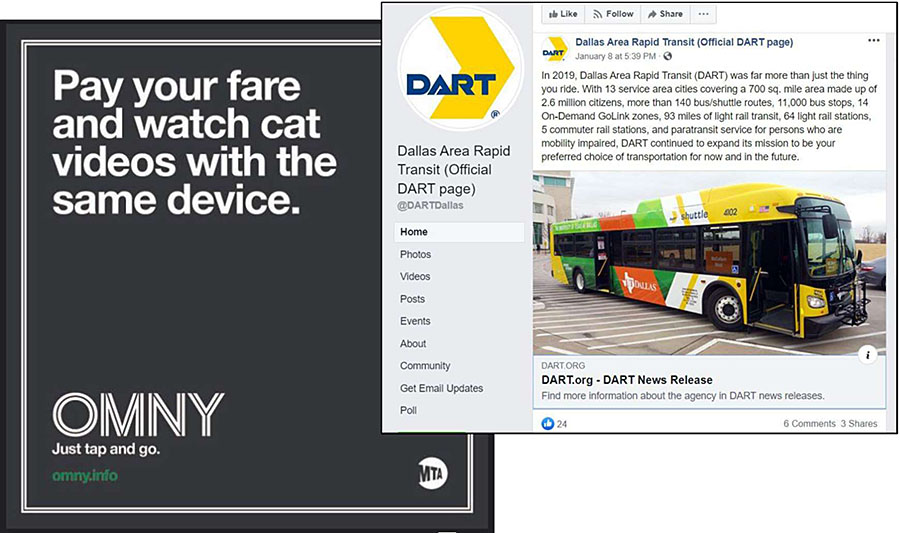
Slide 43:
Customer-Generated Social Media
Overview
- Social media posts from transit customers, stakeholders, and others can provide unfiltered feedback
-
User-generated posts typically include the following
- Questions (e.g., where is the bus? what is the fare?)
- Complaints (e.g., service, maintenance, safety, security)
- Compliments (e.g., operator commendations)
- These inbound communications can be generated by riders, stakeholders, and community members and shared widely.

Slide 44:
Customer-Generated Social Media
Customer Questions
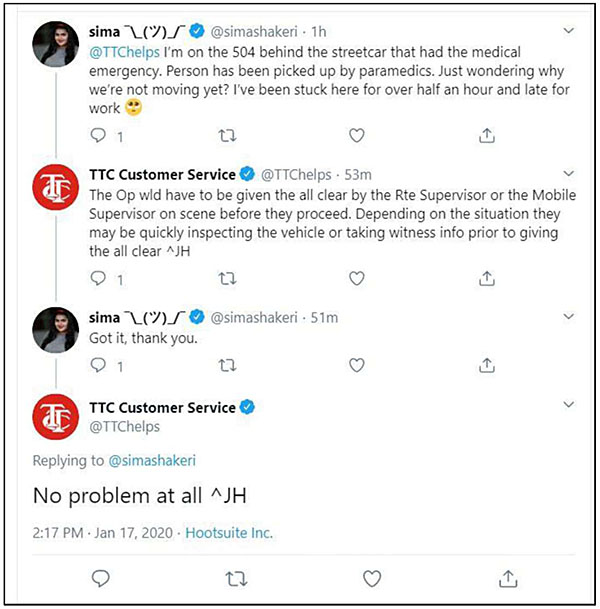
Slide 45:
Customer-Generated Social Media
Customer Complaints
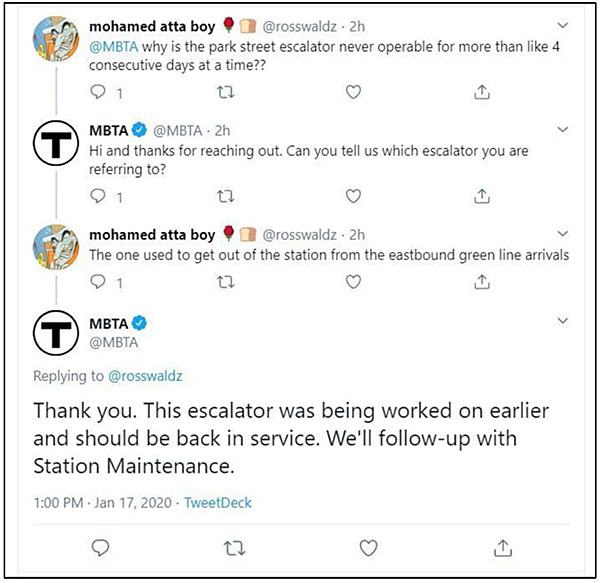
Slide 46:
Customer-Generated Social Media
Customer Compliments

Slide 47:
Crowdsourcing and Peer-to-Peer Communications
Overview
- Crowdsourcing solicits ideas and feedback on a specific topic from a large group of people via the Internet.
- Some mobile applications create a platform for subscribers to share information with one another.
Slide 48:
Crowdsourcing and Peer-to-Peer Communications
Overview
- Crowdsourcing solicits ideas and feedback on a specific topic from a large group of people via the Internet.
- Some mobile applications create a platform for subscribers to share information with one another.
-
Examples include:
- Transit - Mobile app complements real-time data feeds with crowdsourced info
- Pigeon - Google app for crowdsourced info
- Clever Commute - Mobile app for sharing customer info for NJ Transit, LIRR, MNR services
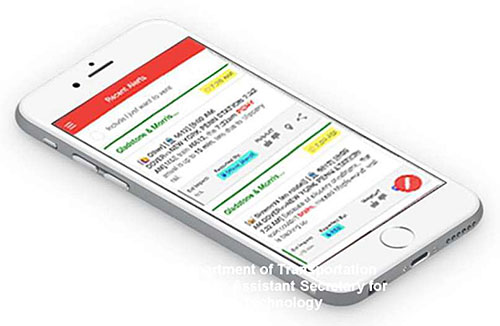
Slide 49:
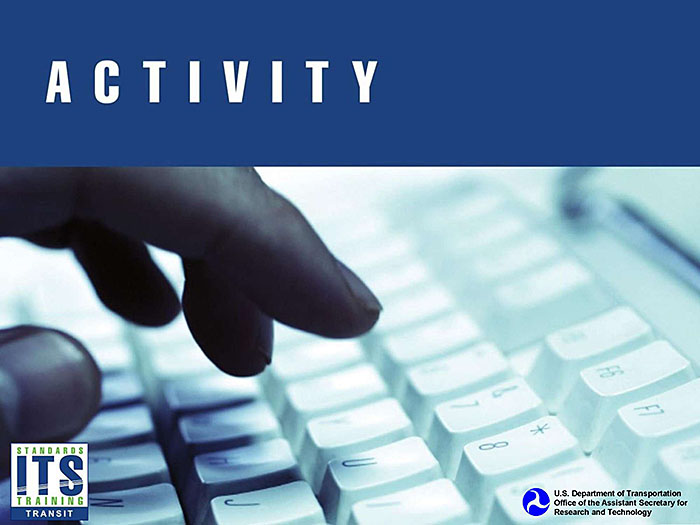
Slide 50:
Question
Which of these is NOT a source of social media data for business intelligence?
Answer Choices
- Agency marketing posts
- Customer complaints
- Customer questions
- Peer-to-peer communications
Slide 51:
Review of Answers
a) Agency marketing posts
Correct! Marketing social media posts can generate goodwill for an agency, but they are not used to inform data-driven decisions.
b) Customer complaints
Incorrect. Customer complaints can provide valuable data.
c) Customer questions
Incorrect. Customer questions can provide valuable data.
d) Peer-to-peer communications
Incorrect. Peer-to-peer communications can provide valuable data.
Slide 52:
Learning Objective 3
- Define big data in relation to social media transit
Slide 53:
Overview
- What is big data?
- Large datasets characterized by variety, volume, and velocity
- Sources of transit-related big data include internal and external data sources
- Characteristics of social media datasets
- Social media data standards are emerging
Slide 54:
What is Big Data?
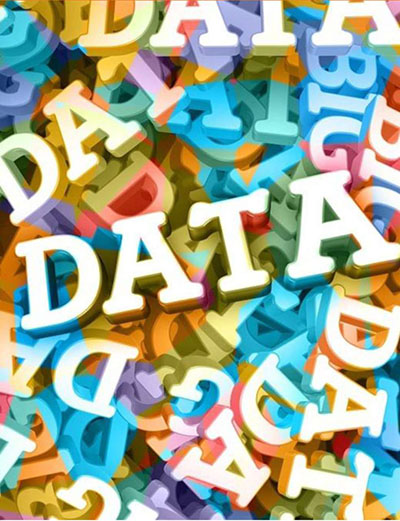
-
Large volume of data
- Structured data
- Unstructured data
- Difficult to process with traditional database and software techniques
Slide 55:
Characteristics of Large Datasets

Large datasets are characterized by their variety, volume, and velocity
-
Variety
- Multiple sources
- Multiple formats: text, photo, video, PDF, database, CSV, spreadsheets
- Structured and unstructured
-
Volume
- Terabytes (1012)
- Petabytes (1015)
- Brontobytes (1027) and upwards
-
Velocity
- Speed required to convert inputs into outputs
- Streaming, which is continuous conversion from inputs to outputs
Slide 56:
Examples of the 3 Vs and Transit-Related Data
Data Description |
Variety |
Volume - Storage |
Velocity -Frequency of updates |
Vehicle Location 100,000 trips per year |
Structured |
3.6 GB per year |
50 bytes per vehicle every 5 seconds |
Schedule Data (e.g., SEPTA bus) |
Structured (GTFS) and compressed |
21 MB |
Seasonal |
Video from 300 Cameras |
Video |
1.2 TB |
Streaming |
Geographic Information Files (NJT Bus) |
Structured |
40 MB |
Seasonal |
Slide 57:
Transit-Related Big Data Includes Internal and External Sources
-
Internal sources
- Rider surveys and panels
- Focus groups and stakeholder interviews
- Automatic passenger counting data (APC)
- Automated vehicle location data (AVL)
- General Transit Feed Specification files (GTFS/GTFS-rt)
- Electronic fare payment system datasets (EFPS)
-
External data sources
- Social media posts
- Census files and other public datasets
- Traffic data
- Web pages (HTML)
Slide 58:
Characteristics of Social Media Datasets
- Unstructured text, written in natural language
- Uncategorized
- Voluminous
- Variety of formats (e.g., JPG, GIF, MP3, MP4)
Slide 59:
Social Media Data Standardization Challenges
Standards may be emerging, but standardization is a challenge.
- Social media is unstructured and may include natural text, images, and video.
- Social media platforms are mostly owned by private for-profit entities and data (e.g., posts) may use a proprietary format.
- Some social media have Application Programming Interfaces (APIs) for downloading data, but others have no API.
Slide 60:
International Efforts on Big Data Standards (1 of 2)
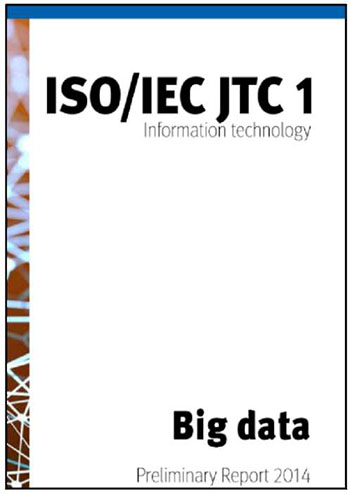
SDO/Consortium |
Interest Area |
ISO/IEC JTC 1/SC 32 |
Data management and interchange, including database languages, multimedia object management, metadata management and e-Business. |
ISO/IEC JTC 1/SC 38 |
Standardization for interoperable Distributed Application Platform and Services including Web Services, Service Oriented Architecture (SOA), and Cloud Computing. |
ITU-T SG13 |
Cloud computing for Big Data. |
W3C |
Web and Semantic related standards for markup, structure, query, semantics, and interchange. |
Slide 61:
International Efforts on Big Data Standards (2 of 2)

SDO/ Consortium |
Interest Area |
Open Geospatial Consortium |
Geospatial related standards for the specification, structure, query, and processing of location related data. |
Organization for the Advancement of Structured Information Standards |
Information access and exchange. |
Transaction Processing Performance Council |
Benchmarks for Big Data Systems. |
TM Forum |
Enable enterprises, service providers and suppliers to continuously transform in order to succeed in the digital economy. |
Slide 62:
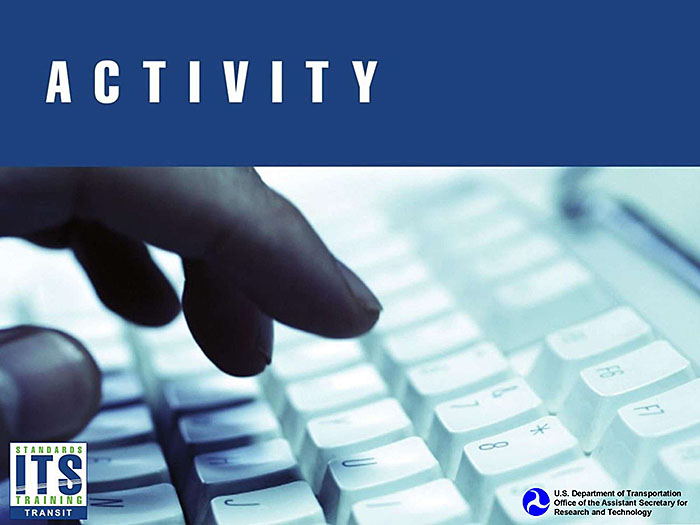
Slide 63:
Question
Which of the below is not one of the 3 V characteristics of big data?
Answer Choices
- Velocity
- Viscosity
- Variety
- Volume
Slide 64:
Review of Answers
a) Velocity
Incorrect. Velocity refers to the speed required to convert input data into output data.
b) Viscosity
Correct! Viscosity is not one of the 3 Vs of Big Data, but a useful measure for assessing the quality of maple syrup and ketchup.
c) Variety
Incorrect. Variety refers to the diversity and inconsistency in the structured and unstructured data present in Big Data.
d) Volume
Incorrect. Volume refers to the quantity of data and growth rate.
Slide 65:
Learning Objective 4
- Understand the process for applying big data analytics to social media to inform transit business intelligence.
Slide 66:
Overview
- Data acquisition
- Data preparation
-
Data analysis
-
Data presentation
-
Other Issues
- Policy issues
- Technical issues
Slide 67:
Data Acquisition
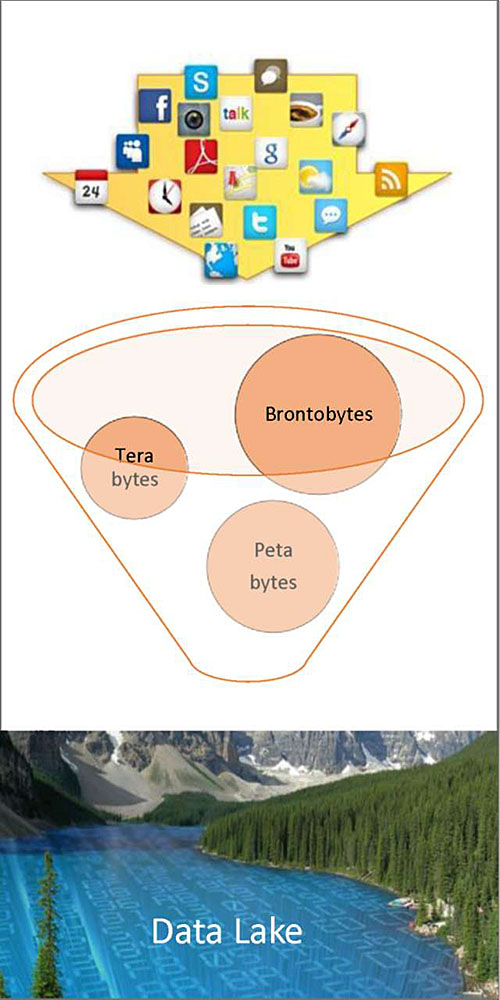
-
Data acquisition is the means necessary to gather data for subsequent steps. These may include:
- Data collection
- Data recording of natural events
- Data recording of human-made events
- Data entry
-
What data do I have?
- Internal sources
- External sources
- What data do I need that I don’t have?
-
Do I need:
- To do data scraping
- To use an Application Programming Interface
- How much will new data cost me to acquire?
-
What are my storage requirements
- Volume, security, in the cloud, in-house
-
Where do I store my data?
- We introduce the term "data lake"
Slide 68:
Data Preparation
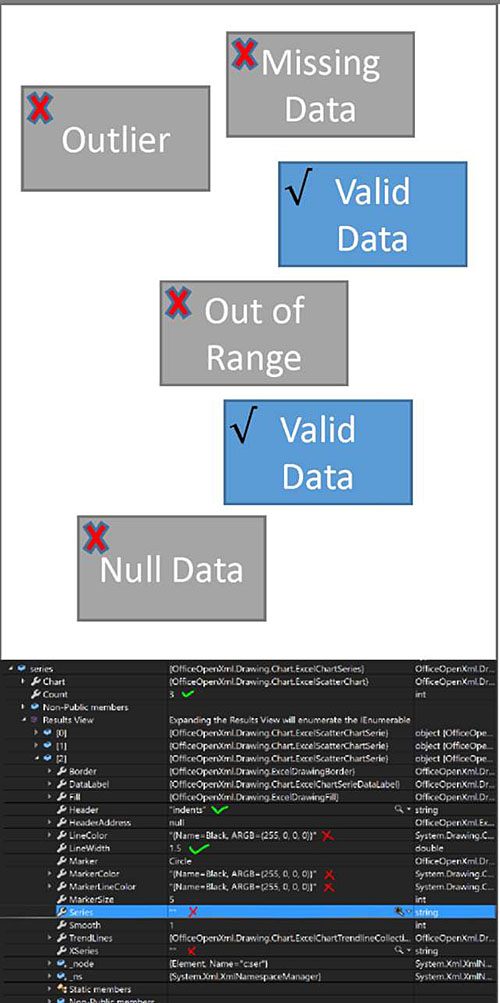
- Data preparation removes data that is incomplete, incorrect, or out of range from analysis.
-
Do I have the right data?
- Granularity
- Coverage
- Content
- Geographic region and data (GPS, GIS files)
- Time frame
- If there is a standard available, this is the step to map data to the standard
-
Data scrubbing and filtering occurs in this step
- Remove outliers
- Handle of missing data
- Remove out of range data
- Handle null data values
- Define any rules for sentiment analysis, topic maps, and linkages between disparate data sets.
Slide 69:
Data Analysis

- Data analysis is the interpretation of relationships between data to gain insights about a problem or solution.
-
Data analysis techniques include
- Data mining
- Data visualization
- Topic maps
- Sentiment analysis
- Data similarity analysis
- Stochastic analysis
- Data correlation
-
Artificial intelligence and machine learning
- Image processing
- Facial recognition
- Automated license plate recognition (ALPR)
- Predictive analytics
Slide 70:
Data Presentation

- Data presentation is the process of using the results of analysis to provide an explanation or make a claim about the data.
-
Agency dashboards draw data from multiple sources to share key performance indicators:
- Ridership
- Service performance
- Financial
- Customer satisfaction
- Maintenance records
- Electronic fare payment

Slide 71:
Big Data Process Steps Summary

Slide 72:
Data Presentation Example: MBTA Dashboard
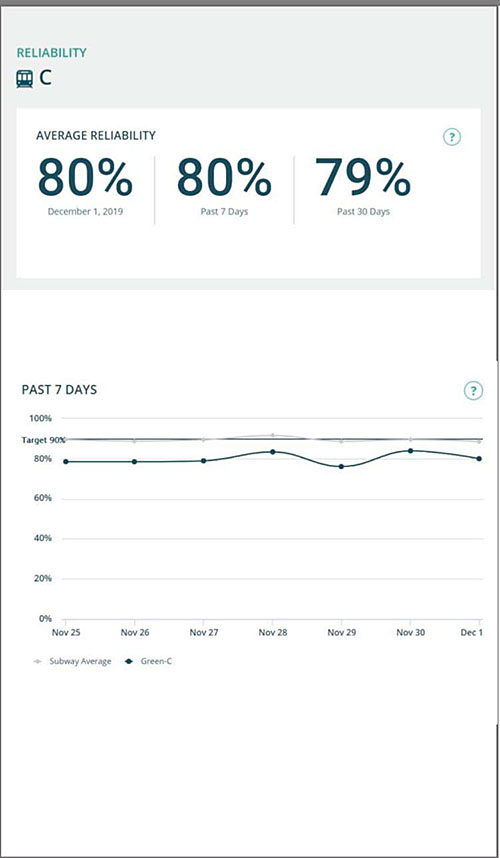
- MBTA has an online dashboard for key performance indicators
- Supports transparency in reporting for internal and external audiences
- Supports drill down by mode, line, and route to get a snapshot of service performance.
- This dashboard does not include social media posts.
- The URL is in the Student Supplement.
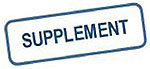
Slide 73:
Data Presentation Example: busstat.nyc

- busstat.nyc measures and displays performance for New York City buses.
- Project is in beta as of January 2020.
- Proposed metrics join data from multiple sources to generate performance indicators that reflect customer experience and agency progress toward meeting goals.
- Route lateness factor compares actual trip time to scheduled trip time. No social media posts were included.
- Project developed by the NYU Center for Urban Science and a capstone project of the master program sponsored by TransitCenter
- The URL is in the Student Supplement.
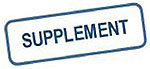
Slide 74:
Other Issues
Policy Issues
- Protecting user privacy
- Data security
- Regulatory environment and limitations/policy of government agencies use of social media
- Understanding how well social media data represents agency customer base
- Analyzing social communications in multiple languages
Slide 75:
Other Issues
Technical Issues
-
A data lake may be partitioned into "data ponds" to:
- Limit access
- Share data resources with another agency
- Provide a means of data sharing between agencies.
- A regional lake may provide ponds for separate transit properties
-
Open source/open data tools
- Need to consider whether adequate technical support and security are available
- Resource requirements (e.g., skills, storage, hardware, licensing, in-house vs. contracted)
Slide 76:
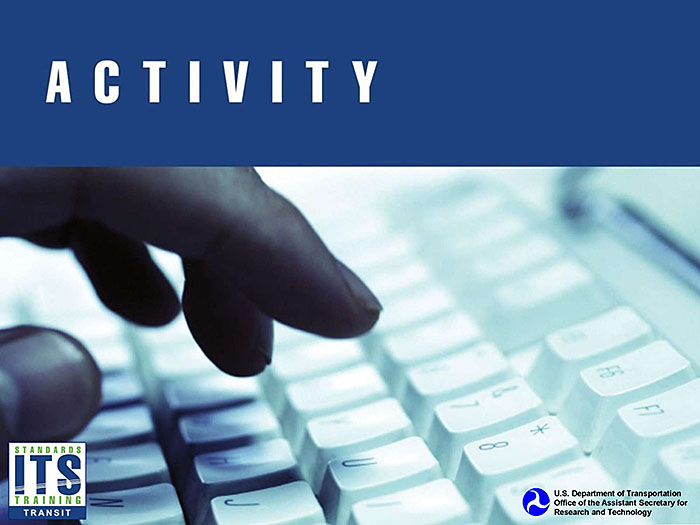
Slide 77:
Question
Which of the below is not a step described in Big Data processing?
Answer Choices
- Data Preparation
- Data Field Quantization
- Data Analysis
- Data Acquisition
Slide 78:
Review of Answers
a) Data Preparation
Incorrect. Data preparation is the step of removing data that is incomplete, incorrect, and/or out of range from analysis.
b) Data Field Quantization
Correct! Data field quantization evaluates elements of the General Relativity Theory to prove gravity exists, and is the basis for the general rule that buses will roll instead of fly.
c) Data Analysis
Incorrect. Data analysis is the interpretation of relationships between data to gain insights about a problem or solutions.
d) Data Presentation
Incorrect. Data presentation is the process of using the results of analysis to make a case or explanation about data.
Slide 79:
Learning Objective 5
- Incorporate findings to support business intelligence with data-driven decisions
Slide 80:
Overview
Working with Social Media
- Social media posts from transit customers, stakeholders, and others (inbound communications) can provide unfiltered feedback.
- Social media posts use natural language, which requires special analytical techniques to create meaningful datasets.
- Posts usually include usernames, which must be removed during analysis to protect privacy.
- Some transit agencies restrict use of social media by staff.
- Social media users may not be representative of all transit customers.
Slide 81:
Overview
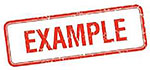
- Chicago Transit Authority (IL)
- San Diego Metropolitan Transit System (CA)
- Transport for London (UK)
- Metro Transit (MN)
Slide 82:
Chicago Transit Authority
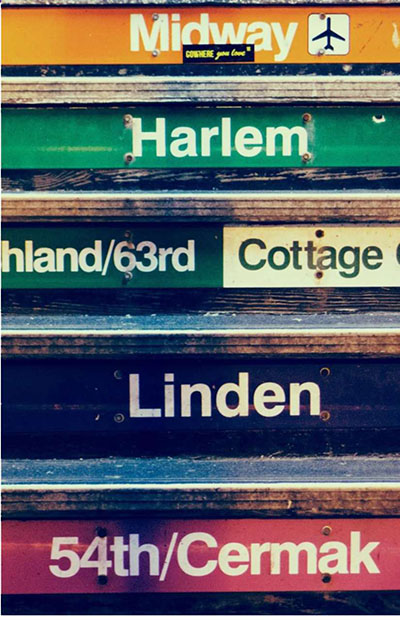
Measuring Customer Sentiment
- In one of the first papers on the topic, researchers analyzed tweets that mentioned the Chicago Transit Authority to better understand customer sentiment.
- Researchers assembled a dataset of Twitter posts that mentioned CTA or individual lines.
- Analysis determined that customers were more likely to express negative sentiments toward a situation than positive sentiments.
Slide 83:
Chicago Transit Authority
Negative tweets spiked at 9 AM on July 23, 2011.
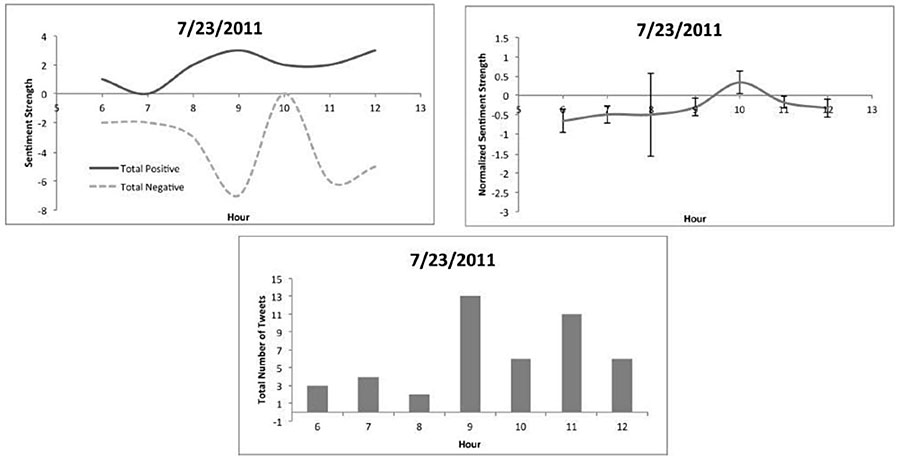
Slide 84:
Chicago Transit Authority
A tag cloud confirmed customer communication around 9 AM about delays on the Red and Blue Lines because of flooding.
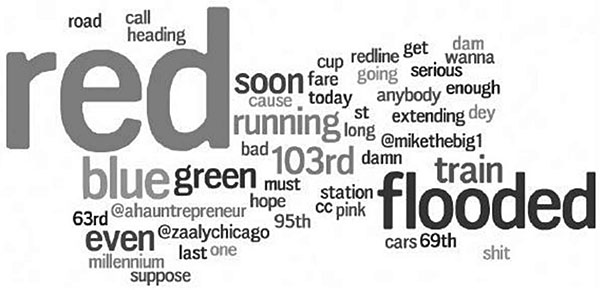
Slide 85:
San Diego MTS
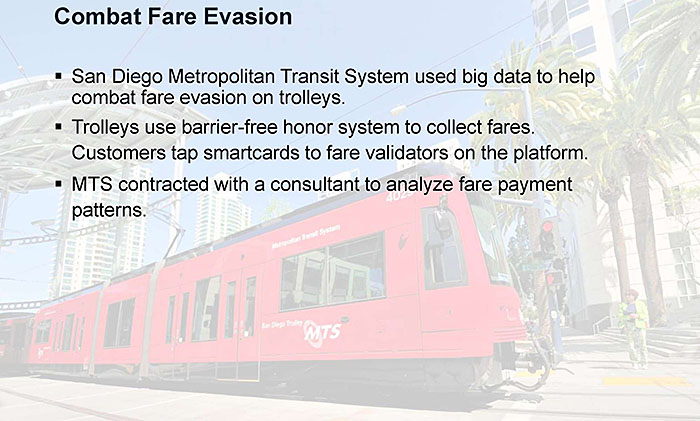
(Extended Text Description: This slide is entitled, "San Diego MTS" with the subtitle, "Combat Fare Evasion." This slide has a background of a red San Diego MTS bus approaching a stop. There are green palm trees near a traffic signal where the bus is stopped. A pedestrian is crossing. Overlaid on top are the following bullet items:
- San Diego Metropolitan Transit System used big data to help combat fare evasion on trolleys.
- Trolleys use barrier free honor system to collect fares. Customers tap smartcards to fare validators on the platform.
- MTS contracted with a consultant to analyze fare payment patterns.
Slide 86:
San Diego MTS
Combat Fare Evasion
-
Analysis incorporated multiple data sources.
- GTFS showed vehicle location.
- Fare validators showed smartcard taps before boarding.
- Automatic passenger counters calculated boardings per station.
- Data analysis correlated farecard taps with passenger counts and vehicle arrivals to determine locations for additional fare enforcement.
- Social media was not a data source for this analysis.
Slide 87:
Transport for London

Optimizing Advertising
- Researchers tested a methodology for analyzing geotagged social media posts in Transport for London Underground stations to optimize advertising campaigns.
- Tweets were analyzed and categorized based on topics of interest (e.g., sports, entertainment).
- Information was intended to provide guidance for advertising campaigns at different stations.
Slide 88:
Metro Transit
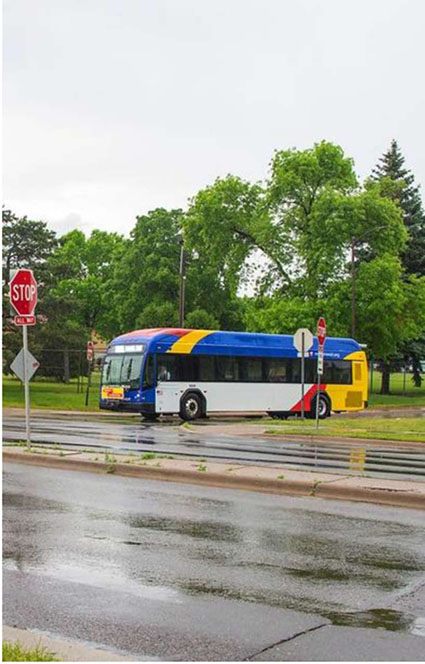
Locating Bus Shelters
- Metro Transit in Minneapolis/St. Paul uses big data analytics.
-
Strategic Initiatives Department draws on data from multiple sources to support data-driven decision making.
- How to allocate resources for bus shelters and amenities?
- How to improve on-time performance?
- How to design a transit network to best meet customer needs?
Slide 89:
Metro Transit

Locating Bus Shelters
-
Data sources
- Customer survey
- Facilities
- Ridership
- Demographics
- Equity-focused measures were developed to inform decisions.
- Data sources do not include social media.
Slide 90:
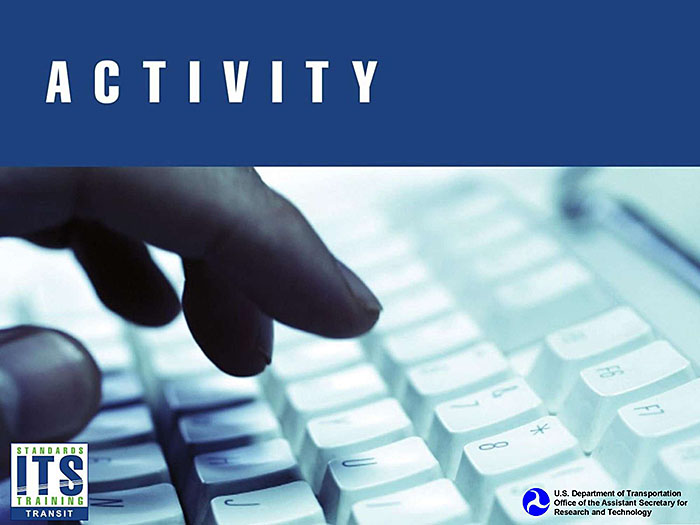
Slide 91:
Question
Based on these examples, analyzing social media data helped inform agency decisions about which of the following?
Answer Choices
- Where to upgrade bus shelters
- How to understand customer sentiment
- Where to add fare enforcement
- How to report non-fare revenues
Slide 92:
Review of Answers
a) Where to upgrade bus shelters
Incorrect. Agency did not consider social media posts.
b) How to understand customer sentiment
Correct! Researchers analyzed social media posts to assess CTA customer sentiment.
c) Where to add fare enforcement
Incorrect. Agency did not use social media to solve problem.
d) How to report non-fare revenues
Incorrect. None of the examples focused on non-fare revenues. Social media is not a source of this data.
Slide 93:
Module Summary
- Learned how transit operators can use business intelligence tools to make data-driven decisions
- Saw examples of agency-generated and customer-generated social media posts
- Learned about potential sources of big data for use in transportation analysis
- Reviewed process for applying big data analytics to social media to inform transit business intelligence
- Reviewed examples of using big data to support business intelligence
Slide 94:
Thank you for completing this module.
Feedback
Please use the Feedback link below to provide us with your thoughts and comments about the value of the training.
Thank you!
↑ Return to top